Predictive Tool: Petro.ai provides for accurate prediction of drainage in a pad and investigates the impact of completions decisions within economic constraints.
Look Back Tool: Petro.ai analyzes data from previous pad production to serve as a planning tool for future production in similar areas within economic constraints.
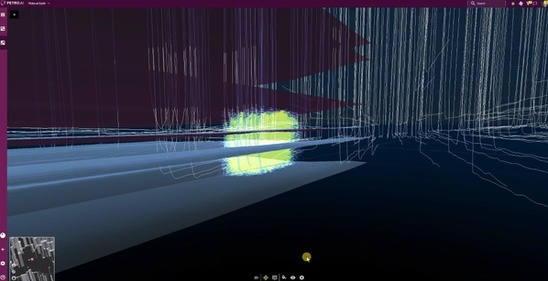
AI Opportunity
The shale challenge is solved by getting geomechanics and AI right.“ Let’s look at the opportunities we exploit with Petro.ai,” Dr. Brendon Hall, VP of Geoscience leads us through the subsurface revealed, “The AI opportunity is dynamically connecting completions, drainage and production. Petro.ai assimilates your conventional approaches into a single computational pipeline.
“We’re dealing with a huge amount of data to get all these models calibrated and working together. It’s difficult to extract that data, clean it and keep it up to date. In Petro.ai, models stay current so that the most recent data you’re getting from the field, which is often the most valuable and the most relevant for the next wells you’re drilling, is brought into the system so decision making has the immediate information needed.
“With that understanding of urgency, Petro.ai has built a single pipeline that keeps those models up to date so you’re making decisions using current models. We’re also leveraging a surrogate modeling approach which means using AI to develop a model of the subsurface that can learn from observations or other physics-based simulations to make predictions. How drainage and geomechanics affect the scope of these drainage areas are instantly associated with your wells’ production and completions activities. That’s the Petro.ai artificial intelligence at work for you, accurate, dependable, and in the right timeframe.”
Geomechanics Opportunity
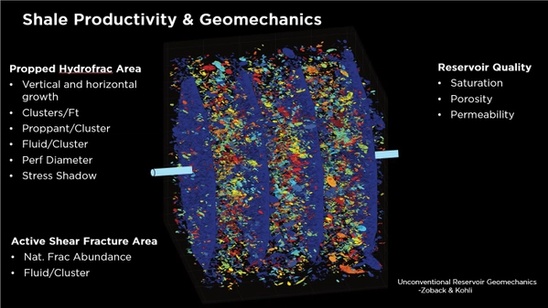
“For geomechanics, we invoke the work of Dr. Mark Zoback, Professor of Geoscience at Stanford University,” Dr. Hall continues. "This is an image from the cover of his textbook, Unconventional Reservoir Geomechanics: Shale Gas, Tight Oil and Induced Seismicity. It shows a numerical simulation of a stimulation job. The blue areas are the propped hydrofracs. All those little colored pieces are natural fractures that have been stimulated by the hydraulic fracture operation. High pressured fluid and sand has gone into the well bore and created those blue fractures. That high pressure fluid is leaking off into the formation causing those fracture plains to fail slightly, by failure meaning to slip. That increases the permeability for a certain amount of oil and gas around that fracture, allowing for seepage into the slippage fracks. This seepage connects back to the hydraulic fracture then to the well bore and gets produced to the surface.
“We’re trying to create these hydraulic fractures, stimulate the rock around them, connect to the natural fracture network in efficient ways so that all the fluids there can be extracted. The reservoir quality speaks to the amount of oil and gas there is available to be extracted. All those pieces have to be in place to have an effective unconventional well. Ideally, we can get measurements of each of these to feed this system to make a prediction about how the well is going to do.
“Petro.ai trains the model by taking those parameters, many of which we’ll know, some we may not. Then we look at what the production was historically to make a prediction about the future.”
Modeling Shale Productivity with AI and Geomechanics
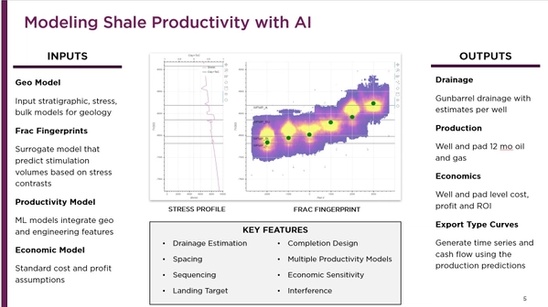
“The slide above is an overview of the Petro.ai approach and how we model shale productivity with AI,” Dr. Hall explains, "On the input side we have the geologic model which includes the layers, horizons, the bounds of the formation that are within the earth. This creates a model of the stress so we know the minimum/maximum horizontal stresses and the vertical stress at each point—the two horizontal stresses being most important for our purposes. And we also include bulk models to include the geology of the reservoir properties.
“There’s the Frac Fingerprint model. This is the AI based surrogate model that predicts the stimulation volumes based on where the completion is in the stress profile.
“There’s a productivity model. This is where we use machine learning to build a multivariate regression model. It can take in a lot of data as features and make a prediction of how much production there’s going to be.
“And also, the economic model. Once we know how much oil production we have, how many dollars does that turn into? Taking all the costs into account.
"On the output side, we output the drainage associated with each well, the production we get from each well, the economics associated with that such as the net present value, rate of return, and whatever KPI we’re interested in looking at. This is at the well and at the pad level. Petro.ai also includes the time series of production. The well’s not going to produce once and then be done. There’s going to be a series of monthly production values that we can predict and decrease over time. We can use that to predict the cash flow.
“This lets us get at an overall estimate of drainage, spacing, sequencing. How far to put these wells apart which we can see from their interacting drainage areas. When to put them in. We can model parent/child effects with this. What landing target is ideal and results in the most productive pad design. How we want to design completions. Look at sensitivities associated with the economic parameters. What effect on economics comes with a variable price of oil?