Digital twin: Petro.ai creates a real-time digital counterpart of shale reservoirs, integrating well data, production, and subsurface data like stratigraphy and geomechanics. We integrate data as it becomes available, so it is always up to date. We have AI models that learn from the digital twin to make predictions of well performance and optimal development plans.
Well-to-Well Interference: If there are existing wells (parent wells) in the area and you drill and complete another one nearby (child wells), when you complete that well and inject all the necessary fluids into the ground and create a fracture, that fracture can travel away from that new child well and impact the stimulated area around the parent well. There can be an effect on production from that parent well. That is what is known as well-to-well interference.
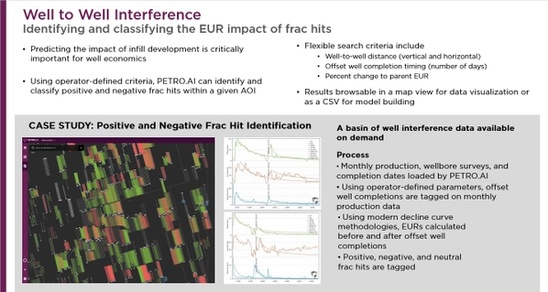
Identifying and classifying the EUR, the ultimate recoverable, of a well lies at the heart of what Petro.ai provides to our clients. What you have to do to get there and the data you have to process are useful not only for this well-to-well interference workflow but for all the information, recommendations and insights. The Petro.ai platform provides this faster and more accurately than any software in O&G today.
“One of the biggest calculations that we do is calculating the spacing relationships between all potentially interfering wells in your acreage,” Dr. Brendon Hall, VP of Geoscience at Petro.ai explains. “The image above shows a grouping of colored polygons and all of those are a spacing calculation that was done between two wells.
“Fractures propagate in the direction of maximum horizontal stress. This we know from geomechanics and our advisor Mark Zoback’s text, Unconventional Reservoir Geomechanics, talks about this phenomenon at length. That’s the direction it’s easiest for these hydraulic fractures to propagate in. And so they do. This calculation is automatically done by Petro.ai. We load up all your wells in an acreage. We run through this calculation automatically. This is a completely automated step with some parameters you can tune for how far around each well you want to search.
“Whenever you add new wells to the system or get new data, this calculation is redone so you have an updated set of spacing statistics at all times.
“These statistics are great features for training machine learning models on whatever you want to train a machine learning model for. We use them as input to our productivity calculators. This can be useful for identifying or being able to predict frac hits or the impact of these interactions on the parent well production. And that’s actually what’s shown here.
“The picture above is from the training set for a well. We also investigate the data and look at the production that was actually measured from these wells. We look at the parent well production when the child well gets completed and we see if there was a positive, negative, or neutral effect. Those are identified by the threshold impact to production. We look to see what the character of the impact on production was. Was it a good impact, was it a negative impact? Did the EUR go down? Or did it return to about normal once the completion job was done for the child?
“That’s what those red and green polygons are showing here. That’s also automatically calculated by the Petro.ai system. Normally this would be a very laborious calculation. For every child well that gets drilled, you have to look at each of its parents later on and line up those production curves. See if there was an impact. Calculate how much and report that. This is automatically taken care of by our processing pipeline in Petro.ai.