2015: Thor leaves his Megingjoro belt (which boosts his power, depending on the source, anywhere from 2x to 10x his usual level) with Tony Stark for safe keeping. Thor took his hammer with him.
2018: Operators discover the parent/child problem. In the early days of shale, the wells were spaced far apart, delineating their acreage. Companies started drilling more tightly and thought they’d get the same production but didn’t.
2021: Operators are suspicious of tools or solutions that have strong use cases in one area but not another. Petro.ai has emerged with an elegant solution that can be applied in different basins based on a two-fold approach: a general aspect that is based on the physics of the rock and then a customized aspect that’s tailored to the basin using specific data types.
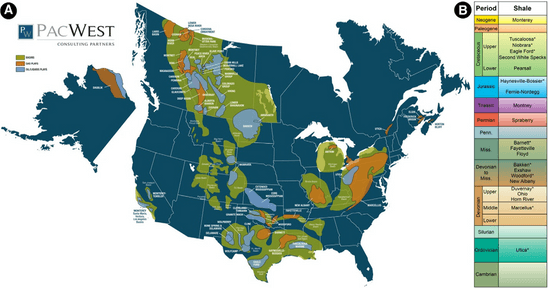
Like Thor’s Belt girdling the North American continent, the oil and gas rich shale basins wait to magnify their already legendary power. How to leverage the costly and challenging rock? Technology. The Fourth Revolution is already on the move in Petro.ai, using machine learning to create predictive modeling and accurate ROI projections for all the basins awaiting the fracking hammer.
World Oil reports that experts are “echoing a widespread view that’s mostly unspoken during the market meltdown: Yes, America can shock the world again. The boom-and-bust cycle will shift, and shale is in a position, with its infrastructure, its ability to ramp-up quickly and its plentiful reserves, to rise from the ashes stronger than ever. When the dust settles in the Permian Basin and other American shale fields, the survivors will be leaner and more tech savvy, according to Daniel Yergin, a Pulitzer Prize-winning oil historian and vice chairman of IHS Markit Ltd.”
And Yergin adds, “Companies go bankrupt, but rocks don’t go bankrupt. When this all shakes out, there will be other people to develop shale.”
And those other people will need a technology that applies lessons learned with the geomechanics and localized data of the high permeability rock. Charles Connell, VP of Product weighs in on Petro.ai’s applicability to any shale reservoir,
“The main challenges and use cases that operators face are comparable across the shale basins. Petro.ai is able to apply a similar approach in the different assets because we tie it back to the underlying stress profile in that area. And because we train our productivity model on data from the identified region. So, it’s a generalized approach that is specifically tailored to an area.”
“The fracture geometry model is trained on data that is local to an asset,” Kyle LaMotta, VP of Analytics continues, “The local part of the geology is also incorporated with that stress model. We’re taking measurements from either the completions data using ISIP’s or a diagnostic data type like a DFIT, diagnostic fracture injection test. And also, some logs. We use clay plus TOC, total organic content, logs as a guide to develop the stress profiles. The stress profiles are explicitly regional to the area that we’re making these predictions in. That’s going to be a big influencer on the direction that the fracs propagate in the vertical direction. Whether they go up or down or make a more oval shape.
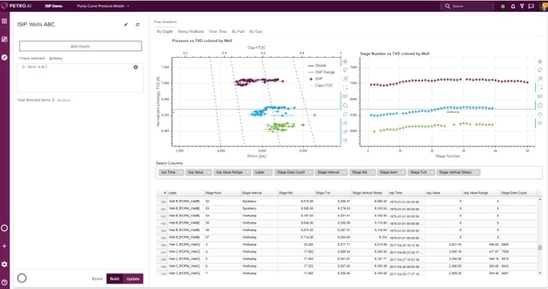
“We have a lot of experience working in all the different shale basins. From a development perspective each one of these basins is going to have different reservoirs that they’re targeting. If you’re drilling in the Barnett, there’s maybe two targets or one. The Eagleford is one target. The Bakken is two targets. When you get to the Midland basin, there could be 5 or 6 targets. The complexity definitely varies across the different basins.”
Connell adds, “In all these different basins, people are trying to understand the optimal way to develop their assets. For Petro.ai, one of our really unique insights is into the vertical stress and how fracs interact, not only horizontally but also vertically and that becomes particularly important when you have more than one target. As Kyle was saying, the Eagleford has one bench and it’s still important to model there to add value where questions of frac geometry still apply.
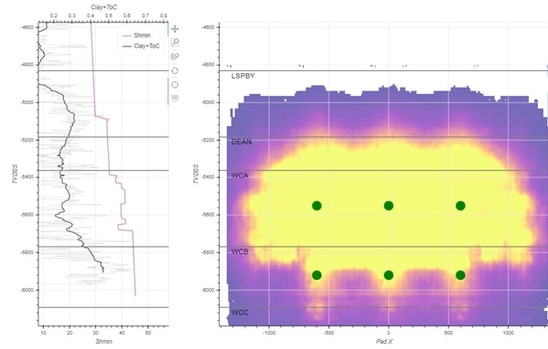
“Now the Permian because it has 5 or 6 layers is a much more complex development process. In these kinds of basins, Petro.ai is vital to address these very complex interactions between parent wells that have been on production for a long time and child wells that you’re going to be putting in which could be interfering both vertically and horizontally.”
“One of the key differentiators is that we also think about the drainage problem,” LaMotta clarifies. “Part of what we’ve built is a multivariate model. There are other companies that are doing that but they’re not able to get the high level of accuracy because they’re not thinking about how much reservoir you’re actually contacting through your frac job.
“One of the variables that we’re calculating through the deep learning approach is the drainage volume. We’re modeling the fracture geometry for each well and then along that lateral we can get the volume of rock that’s being contacted from that completion job.
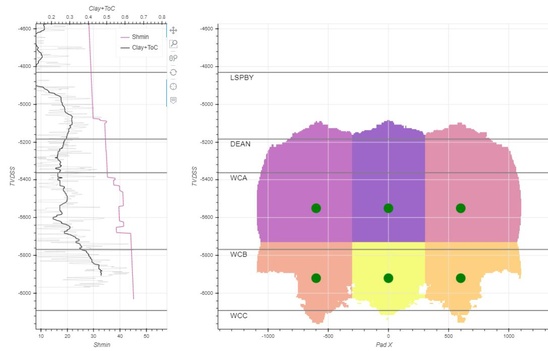
“That’s going to be the same for all shale, no matter what basin. That’s a physics problem, breaking rock. The fracture geometry model is transferrable between these different basins. But the productivity model, that’s the one where we tailor it to a specific area. We’re training the model on the wells around a given area. Then we add in the drainage volumes that have been calculated from the frac geometry model.
“We have the physics side with the frac geometry model then the production side which is the machine learning, multivariate model and that’s taking the local knowledge and the local data around the wells and applying that.
“With those two models we’re taking into account a lot of the key variables that are hard to quantify otherwise. Everything from the geology and the rock properties up to operational sequencing and everything in between like parent/child, spacing, depletion.There are all these variables that an operator has to consider. And the importance could vary across the region. We abstract away a lot of that with these two machine learning models that make it a very accurate way of predicting production.”