Petro.ai: Increase Productivity
Petro.ai: Improve Pad Design
Petro.ai:Consistent Documentation
Petro.ai: Continuous Learning
You’re bringing Petro.ai into your company knowing that productivity will increase, pad design will improve, documentation will be consistent and there will be an ongoing and continuous learning process. How are those goals accomplished? Charles Connell, VP of Product, explains the basics of the steps taken to create your models and recommendation reports. Besides your data, Petro.ai brings in your observational insights and current modeling. Your learnings are injected at each appropriate point in the well lifecycle.You’re the expert. You know your reservoir. Petro.ai is here to digitize and animate that knowledge.
Connell starts with a description of the steps that Petro.ai goes through to validate the modeling, cycling through these steps to refine and tune the model. From that process the Recommendation Reports are generated, “A good way to think about this model is as a Digital Twin of the reservoir. This Digital Twin allows you to ask questions, interrogate the model, try out different scenarios. In order to have confidence in that model and the results, there are a lot of validation steps that we do.”
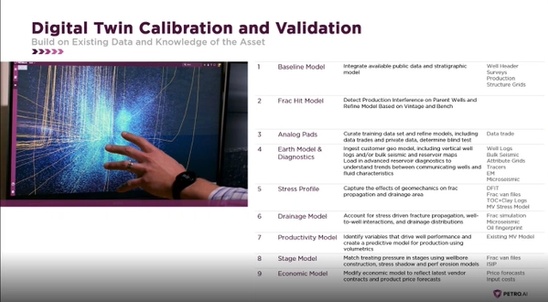
“In the Baseline Model,” Connell continues, “we integrate the data and create the initial benchmark. We take a look at overall trends and QC to make sure everything looks good. Once we get the data in there, we perform key steps in our data pipeline to establish a baseline for all the wells in the area.
“The next step is getting down to the drainage and Petro.ai does this in the Frac Hit Model. Here, we examine the well-to-well interference, detecting parent/child/sibling relationships. We’ll also dial in the sensitivities that are important to you.
“With step three, we curate the training data set and refine models to create Analog Pads. This is where we decide how to manage the blind test which will create an important benchmark that’s tracked throughout the process. This will keep improving as more data is ingested.
“From there we ingest the customer’s geo model and reservoir map information to build the Earth Model and Diagnostics. The data we bring in, working side by side with your team includes well logs, bulk seismic, attribute grids, tracers, EM, and microseismic. We make sure the data driven process is filled out by using your data and public data if necessary.
“The Stress Profile is an important capture of the geomechanical effects on frac propagation and the drainage area. We’ll dig into DFITs, the ISIPs, Frac van files, TOC + Clay logs and MV Stress Model to refine the stress profile.
“The Drainage Model comes out of stitching the data and models together accounting for stress driven fracture propagation, well-to-well interactions and drainage distributions.
“You may have productivity models that you want to use or some key features that you’re interested in to help create the Productivity Model. Otherwise, we’ll go through and look at what’s available in the data set and tune this model to improve the accuracy. Your data and models drive the accuracy of the Digital Twin and our experts using this process help deliver ways to improve.
“With the Stage Model, we look at the frac van data files that you have available and/or if there are perf erosion and stress shadow effects. That allows us to play with some very specific completions data types to tune in on limited entry KPIs. This is a model we’re currently building and training to further improve the accuracy of the digital twin.
“Then we layer on the economics that is intimately tied to the subsurface model to build the Economics Model. Here, we’ll get feedback from your team for price forecasts and input costs that you’ll want to use, modifying the economic model to reflect latest vendor contracts and product price forecasts.”
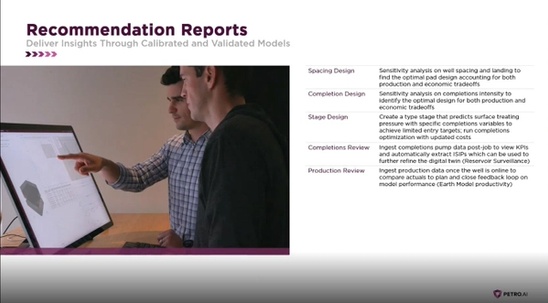
“Once you feel confident with the calibration of the Earth Model (greater than 85% is what we’re comfortable with and we can adjust to your discretion), we’ll create, the Recommendation Reports:
Spacing Design: Sensitivity analysis on well spacing and landing to find the optimal pad design accounting for both production and economic tradeoffs
Completion Design: Sensitivity analysis on completions intensity to identify the optimal design for both production and economic tradeoffs
Stage Design: Create a type stage that predicts surface treating pressure with specific completions variables to achieve limited entry targets; run completions optimization with updated costs. Look at possible perf erosion effects
Completions Review: Ingest completions pump data post-job to view KPIs and automatically extract ISIPs which can be used to further refine the digital twin (Reservoir Surveillance). This helps us tune the model for the next job.
Production Review: Ingest production data once the well is online to compare actuals to plan and close feedback loop on model performance (Earth Model productivity)
“We use the completions review and the production review to improve the digital twin and to better understand the learnings from that pad. Also, we can look at pad options at any point. We can do a hypothetical analysis and provide model variations with their tradeoffs.”
Petro.ai takes not only your data, but your models and your own observations and enters that into a new type of format providing you with a complete understanding of the available matrix of decisions that can be made at each part of the well lifecycle. These recommendation reports based on data-driven, complex calculations layer the economics on the production, layer the economics on the completions. They give you decision possibilities while you dial in the sensitivities.