DSU Design is the connecting glue between workflows in the asset team. You’re doing it all the time: calculating parent-child degradation, well spacing, targeting, completion intensity, handling economics, changing subsurface assumptions. Dropping one well is a $10M decision. We’ve focused our technology on making this a smooth process to share, combine, and interpret from a single view of the reservoir, including drainage patterns.
Machine Learning (ML) models are critical tools in understanding productivity drivers and predicting DSU performance, but including too many features in your ML models reduces their impact on explain-ability and generalize-ability.
At Petro.ai we’ve developed calculation routines that consider your own private data, subsurface characterization, and drainage assumptions to produce customized ML features.
When you use Petro.ai, you can reduce the number of features going into models and supplement your custom features with our own. The result? Improved DSU prediction accuracy and increased trust in financial forecasting.
Let’s first look at the impact of putting in too many features. Sometimes we hear these called “columns,” but in the end it’s the data we send into the ML model. Different ML models are good at pulling out patterns in data sets, and so the ML model you choose is important.An increasingly popular choice is xgboost. Regardless, all models are negatively affected by too many columns. In the training process for all ML models, they will try to find signal from the noise. Any extra information makes it harder for the machine to identify the appropriate signal.
It's a lot like being in a crowded restaurant and trying to hear the conversation across from you. There could be a rowdy table that makes it hard to carry on your more intimate dialogue. The signal you are trying to focus on gets choked out by such a rowdy - but clear - signal.
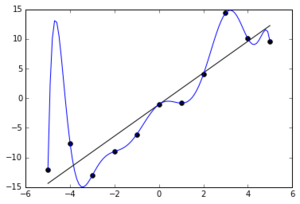
Machines get confused, too. And they will try to associate an outcome – like 12-month production – to the clearest signal it can find. If that clear signal isn’t well associated with the outcome, the machine will weave in other features and try to minimize the residuals, or error, in the training set.
This all puts the machine in dangerous territory. While the training set patterns can be accounted for, there will be little to no ability to understand why those decisions were made.
This puts the model in a precarious position when it is asked to extrapolate the results into the future or a separate AOI.
ML models can give you surprisingly wrong correlations when they are overtrained on extra data.
One example we’ve seen is a model suggesting that increasing HCPV has a negative effect on productivity. Here, the model has clearly extrapolated a model based on noise rather than signal. It would be difficult to earn credibility with experts and gain broad adoption with models like these.
At Petro.ai, we deliver a new set of features that consider the first-order effects driving productivity. Critically, this includes subsurface data types like formation tops, drainage parameters, and well logs. We merge those characterization products of your team to produce a list of features that deliver clearer information to the machine. In our dinner example, we move your conversation to a private room, buffered by walls, delivered by Petro.ai.