Best Paper Award – URTeC 2022
More than 190 technical papers were presented at the 2022 Unconventional Resources Technology Conference (URTeC). Of this elite group, only 9 papers were choses as the “Best of 2022” by the URTeC Awards Committee.
Petro.ai was very proud to be recognized as an author, alongside Technical Advisor Dr. Mark Zoback, as the Best Paper in the Rock and Hydraulic Fracture Modeling theme.
The paper, Lithologically-Controlled Variations of the Least Principal Stress with Depth and Resultant Frac Fingerprints During Multi-Stage Hydraulic Fracturing, introduces the concept of a Frac Fingerprint and introduces case histories of Frac Fingerprints being used to choose optimal well spacings, landing depths, estimate drainage volumes, and ultimately forecast production.
A key insight of the paper is the fact that horizontal and vertical hydraulic fracture growth is chiefly governed by the variations in the magnitude of least principal stress above and below the fracture stage.
Complex, multi-layered viscoplastic variations in least principal stress are parameterized using machine learning techniques. This method of creating a continuous profile is a significant advance beyond the current practices based on the Eaton equation. These models underpin Petro.ai’s ability to accurately quantify and forecast PUD well fracture geometries, drainage patterns, parent/child interactions, co-developed sibling well effects, and well productivity.
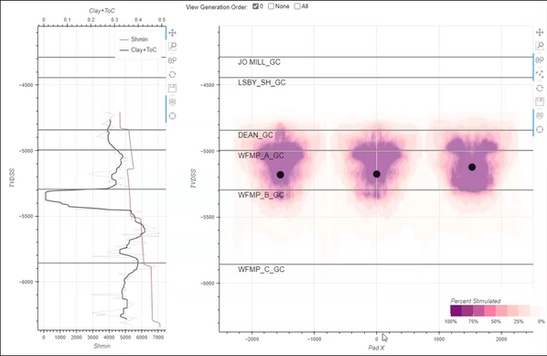
Upcoming Papers – URTeC 2023
To follow on the success of 2022, Petro.ai will be presenting 3 technical papers at this year’s event (URTeC 2023). These papers combine the Frac Fingerprint concept introduced last year in three distinct AI/ML use cases: child well degradation models, optimizing development sequencing, and inventory valuation.
Optimizing Shale Infill Development with AI: Child Well Degradation Models
Optimization of a drilling spacing unit (DSU) with parent wells, or even the accurate forecast of infill wells, is hindered by the large degree of uncertainty around child well degradation. Infill developments have seen up to a 40% degradation in the Midland Basin. Petro.ai demonstrates an AI workflow to predict infill well performance and determine optimal well placement that is applied to a five square mile development in the Midland Basin. This workflow uses a flexible approach that accounts for various attributes influencing child well performance such as offset distance, cumulative production, or other features. Engineers are able run design sensitivities on well count and placement to improve the economics of a development while reducing the uncertainty in their production forecasts.
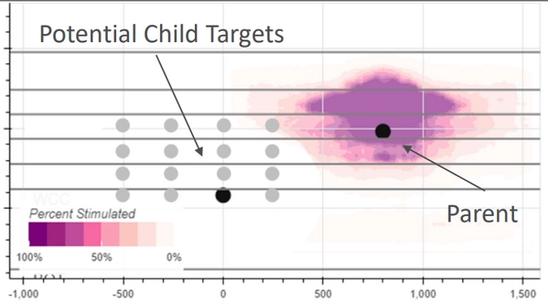
A ML Workflow to Quantify the Impact of Development Sequencing and Parent-Child Interactions with a Case Study from the Delaware Basin
The sequencing of development, across a drilling spacing unit (DSU) and across the various target intervals, creates wells with the potential to underperform analogous, unbounded wells. These parent-child interactions make accurate production predictions difficult and hurt the economics of the DSU. A physics-informed ML workflow is presented that accurately models in-zone and across zone parent-child interactions. A specific example from the Delaware Basin is provided where the workflow was applied to the development of four target intervals. Engineers and geoscientists can apply this workflow to quantify the impact of sequencing decisions so that they can mitigate the impact of well interactions and can more accurately forecast expected production.
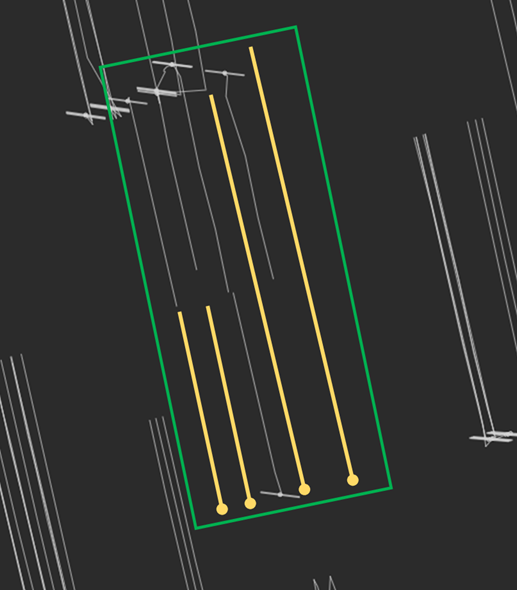
A Subsurface Informed ML Approach to Inventory and Opportunity Evaluation That Supplements Type Curve Workflows
Traditional approaches to development planning break down in areas where few analog wells are available to create type curves. This situation is common on the periphery of basins where well counts fall off, increasing the uncertainty and error in forecasts. A modeling approach is presented that ties well performance to vertical and spatial changes in geology. The approach is applied to a use case in the Delaware Basin where inventory locations were identified and the development sequencing of 24 drilling spacing units (DSUs) prioritized. Accurately predicting and prioritizing inventory locations is fundamental to capital disciple. Using the workflow outlined, engineers are able to accurately forecast wells in areas lacking significant analogs or having rapidly changing geology.
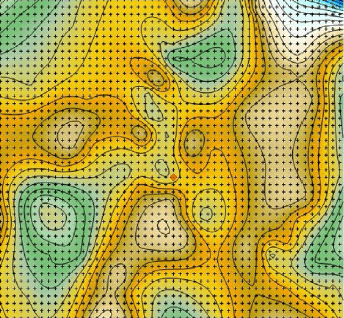