Revolutionizing Well Performance Forecasting with Machine Learning in the Midland Basin
Forecasting the potential of inventory locations in unconventional oil and gas plays has long been a complex challenge. Traditionally, engineers rely on type curves—a baseline curve based on the performance of similar wells—augmented with risk factors to account for geological variations, well design, and offset interactions. However, even with these methods, uncertainty remains, as engineers must manually assess various tradeoffs. Enter machine learning (ML), a transformative tool that’s shifting the paradigm in predicting well performance, optimizing development strategies, and enhancing decision-making.
The Shift to Machine Learning in Unconventional Development
Machine learning’s application in predicting well performance has garnered increasing attention in the oil and gas industry, especially in unconventional resources. Studies such as Zhou et al. 2019 laid the groundwork for using larger datasets to optimize well completion, spacing, and predict locations for potential undeveloped (PUD) wells. More recent advancements, like those by Ren et al. 2023 and Meyers et al. 2021, have further refined workflows, tackling the challenges of applying ML to reservoir predictions and inventory performance. These approaches aim to improve accuracy, reduce human bias, and speed up decision-making by providing data-driven insights.
While many off-the-shelf modeling solutions exist, the real challenge is not just in creating a model but in overcoming hurdles in data collection, curation, and feature generation.
An effective model requires testing for biases, ensuring accuracy, and dealing with large and diverse datasets. That’s where a fully automated machine learning system comes in—streamlining the entire process from data ingestion to model deployment, and enabling rapid experimentation for well production predictions and more efficient inventory planning.
Modeling the Midland Basin: A Case Study
To bring this ML vision to life, consider the Midland Basin—a prolific area with over 17,000 horizontal wells. The PetroAI pipeline models the basin by integrating various data sources, including well data, drilling surveys, and monthly production figures, to generate predictions. Internally interpreted subsurface structure grids and geologic maps are also incorporated, providing a higher level of accuracy than relying on publicly available sources.
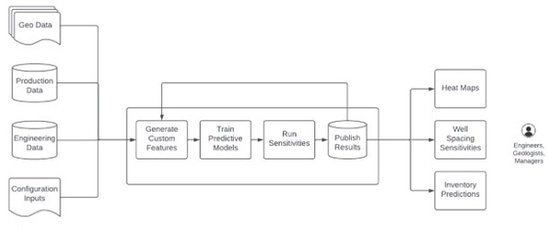
This modeling pipeline automatically processes incoming data, runs feature extraction, and uses a decline curve analysis (DCA) engine for well forecasting. The PetroAI pipeline then segments wells, calculates offsets, and classifies them as parent, child, or sibling wells, while generating a Frac Fingerprint—a crucial step for evaluating well interactions. This process allows for rapid experimentation on various features, enabling the system to produce robust, actionable predictions.
Building Better Models Through Iteration
The strength of this system lies in its ability to quickly iterate and refine models. Over 29 model iterations were run to improve performance and refine predictions. Key geological factors such as proppant loading, lateral length, and total drainage were chosen based on their strong correlation with production. These inputs helped define the cube extraction rate (CER), the target variable for forecasting. Initial results were promising, with a fivefold cross-validation accuracy ranging from 77% to 84%, though some areas with sparse well data required additional tuning.
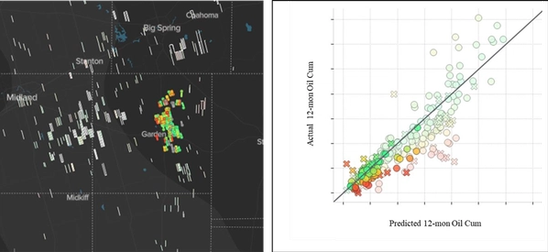
One of the challenges faced during this process was the scarcity of data in certain regions, particularly along the edges of the basin. The modeling team applied a solution by simulating virtual surveys for each inventory location and feeding them into the pipeline for prediction. These virtual surveys allowed for assessing the potential of locations that may have otherwise lacked sufficient real-world data for analysis. This step helped provide more reliable predictions for those "upside" target areas and delivered accurate economic models for each well.
Applying the Models to M&A: A Game-Changer for Decision Making
The true power of machine learning models becomes apparent when applied to high-stakes scenarios like mergers and acquisitions (M&A). In one such case, a previously un-marketed opportunity along the edge of the Midland Basin—spanning 100 potential locations—was fed into the pipeline for performance and cash flow predictions. The results revealed a key trend: well performance decreased moving east from the core of the basin, supporting the decision to pass on further exploration.
This quick, data-driven analysis enabled decision-makers to bypass traditional, labor-intensive workflows, cutting down on the time required to evaluate such an opportunity. With models providing fast, accurate predictions, companies can make more informed decisions with greater confidence.
A Scalable Solution for the Future
What’s most exciting about this machine learning approach is its scalability and flexibility. By incorporating unique geological features, well design, and the interactions between parent, child, and sibling wells, it significantly reduces uncertainty in development planning. Looking ahead, this system can be extended to optimize well placement across different target formations, run sensitivities on well spacing, and even incorporate real-time drilling programs into the analysis.
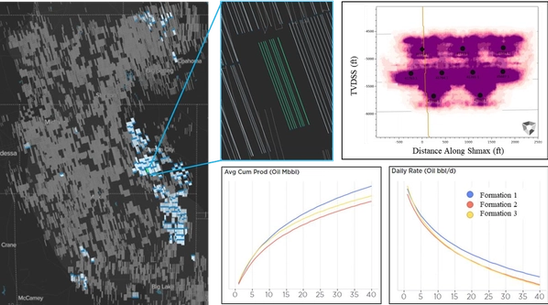
For engineers, this means creating highly accurate forecasts for future wells, improving the overall planning process. Whether it’s adjusting completion designs, assessing the impact of well spacing, or understanding the depletion effects of neighboring wells, the ability to run these "what-if" scenarios allows for more effective, data-driven decision-making. Additionally, this system is particularly valuable for M&A, providing a robust, data-driven forecast for potential inventory locations.
Conclusion
In an industry where decisions often have a significant financial impact, machine learning offers a powerful tool to cut through uncertainty, optimize development strategies, and streamline forecasting. By leveraging advanced data processing, feature extraction, and automated modeling workflows, companies can gain a deeper, more accurate understanding of their reservoirs. As the industry moves toward increasingly complex data environments, machine learning will undoubtedly play a critical role in shaping the future of well performance forecasting and resource development.