with David Cannon, Senior Vice President of Geoscience and Technology at Diamondback Energy
The views expressed here are not the official views of Diamondback Energy, but those solely of the subject of this interview.
If you missed the first part in this series, you can find the start of our conversation here.
What do you see as some of the key technologies that are going to help you succeed in this price environment?
There’s a lot of very cost-intensive technologies out there. During good times in the oil and gas industry, people go out there and spend millions and millions of dollars collecting high-resolution data: microseismic surveys, wide azimuth seismic shoots, pilot holes, logs in the laterals, and so on. This data comes at an extremely high cost and can give you very detailed data from their collection, but during times such as these, they're usually the first items cut, because they're considered discretionary spending. To follow the theme from my first post, the social proof is no longer there. So during poor price environments, we must fall back upon our scientific understand and dust of our mental models to contextualize all that high-end data we collected during the good times.
Pilot projects, high-density data pad projects, a lot of those wellbore centric data collection efforts are really just that—wellbore centric. What does that tell us about a complex reservoir 2 miles away? It doesn't really tell us much. It's hard to take that high-resolution data in a very finite area and extrapolate that to other parts of the reservoir without knowing context.
Geologists utilize many mental models. That's what we do day in, day out. Sequence stratigraphy is a mental model. Structural geology concepts are mental models. Depositional environments are mental models, and we have to take all those data sources that we've collected and put them within that mental model. This is a key step in our progression toward data analytics and machine learning.
In data analytics and machine learning, the algorithms need context. You can't just throw numbers into a black box and expect results to appear that make any physical sense, so you need to contextualize these things, that is where I've seen successes recently. Data science and machine learning are getting better with the contextualization. That has been my biggest issue with data science in the past. Very early on, it was very black boxy. You threw your data in the box, never to be seen again, then a result came out. Well, how did that work?
At Diamondback, we're very curious about that workflow and critically interrogate whoever we partner with that provides these services. We want to see how it works. We want to be able to understand the entire process from data loading to result creation, because if we can’t explain it physically, then there's no point acquiring it. It's just a number, it's just a result at that point, completely unknown with no uncertainty bounds whatsoever. How can I put any trust in that value if I don't understand it? That's where a lot of advancements have been had, and I think will continue to be had, in 2020 and beyond within this particular industry—pushing geologic contextualization within data science constructs.
What successes have you seen with data science or machine learning?
At Diamondback in particular, I think we've run the gamut. We've done some projects around machine learning early on, back in 2016. One of the questions we wanted to answer was:
Here's a whole mess of data on horizontal wells—gamma ray profiles, stimulation designs, post job reports, drilling data. Here's all the geological information, what formation they're in, all this other stuff. And basically, they smooshed it all together and they spit out a couple of answers for what drives production. Those two things? Better production from wells that were toe up and had little undulation. So does the subsurface play a role at all in this? And when we were interrogating the provider on that, they said, “we don't have enough data”.
What does that mean? We gave them 300+ wells to utilize. But it turns out that's not nearly enough data. We needed an order of magnitude more. In that particular example, what really enlightened us was the effect of data population. In order for an algorithm to properly learn through data, you need a ton of it.
If you don't have a lot of data, the learning band that algorithm has is very, very narrow. You do not create an environment for that algorithm to adapt to new inputs, to outlier inputs. If you have a population of 200 wells and you have knowledge that 20 of them have outlier type production, that's 10% of the population. Those outlier results start becoming part of the distribution. That's a problem.
One of the things we see as a success with our partnership with Petro.ai is proper contextualization of a data science project. Tapping into geoscience expertise to build better contextualization, in addition with more data, will result in better outputs. Outputs that we can trust in our business decisions of directing a multi-million dollar capital budget.
It seems like Diamondback has had success with data trades. Do you see that as a trend in the industry or is that sort of unique to what you guys are doing?
No one outside of the majors has the type or volume of data necessary to make a machine learning algorithm output results that are meaningful. The majors have thousands and thousands of wells, which is why they have whole departments dedicated to data science and machine learning for their own internal use. There's a reason why, a lot of times, they don't contract vendors because they have their own data scientists building the algorithms themselves and using them within the mental models at Exxon or Chevron.
For people like us, we have to rely upon outside data sources, either through data trades or incorporating other means of information, and that's one of the things we've been able to do very successfully, acting upon those data trades and squeeze a ton of value out of that data.
One of the justifications I’ve used with management teams for high-resolution data is how this expense can be scaled to how many trades I can make with the data. If it costs $1 million dollars to collect and analyze a core, it will theoretically cost $167,000 because I will trade this data for 5 others cores nearby with competitors. That in turn will allow us to gain more knowledge on our reservoirs without increasing capital cost.
For our industry to continue to move forward as the preeminent source of energy, either stand alone or in conjunction with other sustainable energy sources, we all have to work together. Working with others with different perspectives keeps our innovative skills sharp. If you are insular, you fall into complacency.
We have built a system that's competitive through public trading of companies. We want to compete with Apache, Concho, and Pioneer, but the problem is, we're all extracting the same commodity. It's not like my oil is any different than Pioneer’s oil and it's not like the end game for that particular barrel of oil is any different either. It's still going to get refined into the same suite of products that we all use and enjoy in our modern society.
We need to start combining our efforts to focus on more industry-friendly consortiums that allow us to share not just data, but share ideas. That’s really what it comes down to at the end of the day. If your rock is good, your production is good, full stop, but more efficient ways of extracting could potentially lift areas that maybe have marginal rock to make it economic. That is the power collaboration. We should all be working together to continue our ability to provide energy to our world to lift up the human experience.
Are there any good books or blogs you would recommend?
I'm a huge fan of social psychology; or the study of human interaction. Also, I am a fan of how human interaction and environmental interaction creates the basis for all cultures and social constructs. What are the things that you can that you can learn from interacting with humans that can provide a better basis of doing business with each other? There are a couple authors that I really enjoy reading books from. One is Yuval Noah Harari. He wrote one called Sapiens: A Brief History of Humankind. It’s a real interesting read about how our species evolved and got to the ‘top of the pyramid’. He’s written a few others. One of them is Homo Deus, which is about how our technological adaptation and our ability to communicate on a much grander scale now because of the proliferation of information. This has set into process an evolutionary change within humans that could be permanent. And as we move forward, he believes, this is the time where we may be able to classify ourselves as a species as something different from Homo sapiens in terms of our ability to coordinate with each other and how modern stimuli have changed us mentally and physically.
The other one I’m reading right now is 21 lessons for the 21st century. It’s a very good book. I’m about halfway through it right now. Lot of interesting topical things in there that contextualize modern issues. The other author I really like is Malcolm Gladwell. He's a journalist by profession, but he's a fan of investigating how people interact and push ideas through society. He's a firm believer of outliers, usually the very few within our society that push a majority of our advancements and ideas through society.
How would you describe Petro.ai?
Petro.ai provides a differential product that allows our company to have an edge on understanding what we're doing in the subsurface and how we interact with it.
As we were selling this project internally, I think what differentiates you from other people, is the fact that, simply put, you provide the platform and access to expertise to allow us to thrive within your platform. And that is something that is differential. There's a lot of people who push machine learning, who push their own platforms for being able to come to terms with how we interact with the subsurface, but it's usually all project-based. They don't give you the keys. It's like an Uber. You have to call them and they show up and they pick you up, whereas you guys are selling us the car. We're able to drive it ourselves, but then we go back to the dealership every so often: something's wrong here and you help us fix it, but we still own the car at the end of the day. So that's kind of how I view Petro.ai.
That's the kind of partnership we want, as I said before, we want to understand. We want to be able to know where to fix the issue if something breaks down. That is important to us because it allows our organization to learn and adapt in the proper manner to new information.
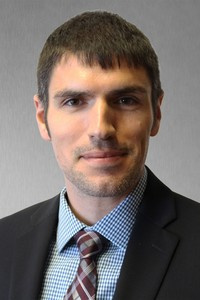
David Cannon has served as Senior Vice President of Geoscience and Technology since February 2019. Previously, he served as Vice President of Geoscience from April 2017 to February 2019, Exploration Manager from March 2015 to April 2017, and as a Senior Geologist since March 2014. Before joining Diamondback, Mr. Cannon served as a Senior Geologist for Newfield Exploration, from January 2013 to March 2014, where he assisted in exploration, assessment, and development of SCOOP/STACK properties in the Anadarko Basin. Prior to that, he held the position of District Geologist for Samson Resources, from August 2011 to January 2013, where he held a position in the Corporate Exploration Department assessing Rockies, Mid-Continent, and East Texas unconventional plays. He was recruited by ConocoPhillips in 2008, where he held various positions in exploration and development of Rockies and Bakken assets. Mr. Cannon received his BS Geology from the State University of New York, College at Brockport in 2005 and obtained his MS in Geoscience from Pennsylvania State University in 2008 with a focus on structural geology and rock mechanics.
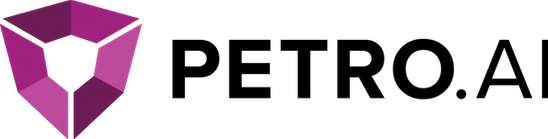
Petro.ai uses AI and Machine Learning to empower domain experts, data scientists, and executives with instant information in the right context, energizing teams and transforming data into action. Partnerships with global leaders in cloud computing (AWS) and geoscience (Dr. Mark Zoback) allow Petro.ai to deliver differentiated technology and teams to Accelerate Discovery.