Q: We have a complicated reservoir, how can geomechanics help?
A: "As we said in last week’s blog, when things get complicated, they’re going to be different all the time,” Dr. Brendon Hall, VP of Geoscience at Petro.ai explains. "It’s important for us to have a systematic way to break things down into different buckets where we use the Petro.ai Drilling Spacing Unit Design Service to analyze section by section.
“The three major systems are geology and subsurface which includes geomechanics, the engineering aspects, and the economic aspects of the development of a DSU.
“There’s a lot of interacting systems that Petro.ai brings to bear that also introduce complexity. We’re interested in how the subsurface geologic parameters affect the engineering aspects of building these systems. The engineering aspects inform the economics. Each system is complex and each of them interacting together makes something that’s even more complex.
“We have an established process for all those systems which is becoming more and more automated. Wrapping our heads around uncertainty is what AI is good for. AI is good for understanding the complex relationships between the various factors, variables and features that are influencing the problem and making sense of those complex interactions. Building the best pad you can given that complexity and uncertainty.
“Subsurface complexity arises because we really don’t know much about the subsurface. We can poke a few holes in it and in the case of shale, we’ve poked quite a few holes in it. Yet, the subsurface is a vast volume and a few thousand wells only begins to describe what’s going on down there. We’re talking about millions of years of earth surface processes that’s given rise to what’s in the subsurface that we’re drilling into. Describing that from a one-dimensional series of points is difficult.
“We’re really trying to use this scarce amount of data to make a prediction of a complex engineering process that involves moving fluids through a very complex pore and fracture network up to the surface in a consistently predictable way.
“What we try to do is characterize the subsurface the best we can. We know that there are some subsurface properties that are important so we can integrate those from data sources like well logs which are the primary source of information about the subsurface. Seismic is another way we can get a picture of these but they’re really low-resolution ways of getting at it.
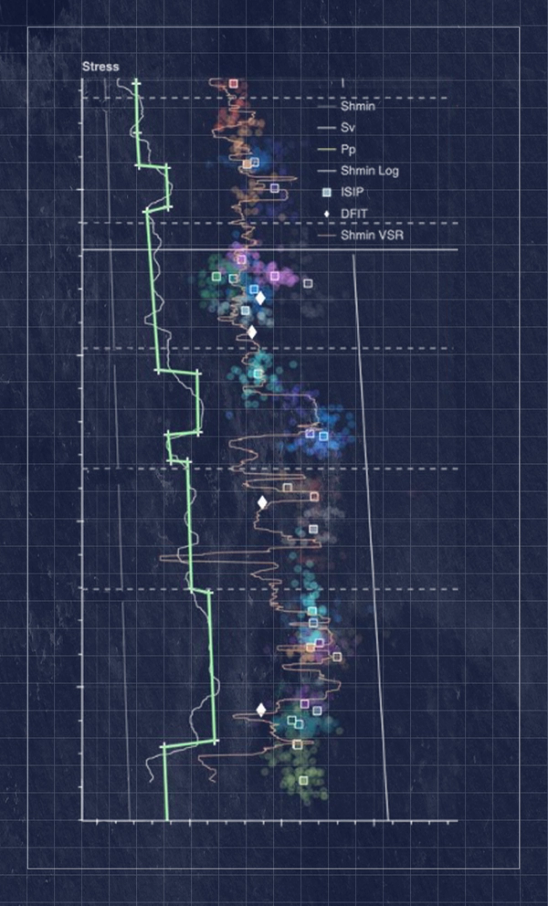
“Something else that’s important for shale is geomechanics. Understanding how the stress changes in the subsurface is really important to know where fractures are going to go, how our wells are going to interact, how we are going to space them, how the wells are going to communicate laterally or vertically.
“We have a process like Viscoplastic Stress Relaxation (VSR) that integrates subsurface properties like well logs in the area. That’s an AI based technique to characterize that.
“We’ll look and see how properties are changing over an area and look at specific subsurface features. For instance, some areas will have carbonates and areas nearby that don’t. That’s the nature of how these things grow. Carbonates are like reefs. They grow up in a little area and are confined so away from that outgrowth you don’t have them anymore. We’ll do an assessment to see if those properties are changing significantly. If they are, we’ll make sure that’s captured in the model. That’s a source of subsurface complexity.
“One of the other things we capture from the subsurface would be petrophysical logs. Petro.ai can run measurements using logs and then do some calculations for oil saturation, porosity, permeability, hydrocarbon pore volume—to develop a spatial understanding of how those things are changing and then extracting those values at the well level. Once they’re at the well level, then we can turn them into features that the model can learn from and predict on.
“We’ve found that when we have those petrophysical properties that are well understood from a large number of logs those tend to be very predictive features that can be used to explain the uncertainty in the model.”