Q: How are the Petro.ai DSU Design Services recommendation reports compiled?
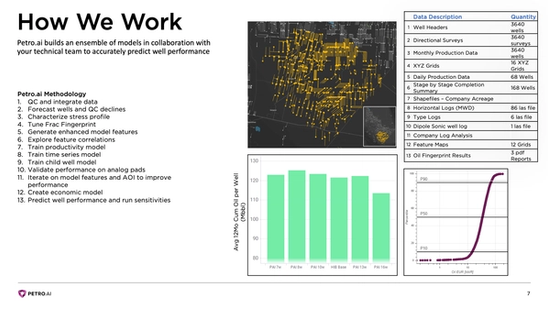
A: “The slide above,” Charles Connell, COO of Petro.ai explains pulling up the power point slide used in the Petro.ai Drilling Spacing Unit Design Services (DSUDS) Kickoff presentation, “outlines the process that we take internally to deliver the DSUDS recommendations to the client. It follows a pretty standard data science workflow. The terminology has been tailored to what we do and the problem that we’re trying to solve.
“We use our internal software that we’ve developed to walk through this methodology. We also apply a lot of our domain expertise along the way. All the people on the Petro.ai internal team that work with our clients have a domain knowledge that they bring to support the software outputs. It could be specific or general. We have Kyle LaMotta and Asal Rahimi who are reservoir engineers and Dr. Brendon Hall and Martin Bentley, who are geoscientists.
“We interface with the entire asset team on the client side. We bring data from each of the different asset team’s domains together to build these models. We walk through this process by first getting our arms around a diverse data set. We’ll talk with completions engineers and bring in different completion data types. Specifically, we’re looking at ISIPs and DFITs that ultimately help characterize the stress profile.
“The Petro.ai team will talk with our client’s geologists and geoscientists and bring in seismic extracted attributes. We’ll bring in different petrophysical well logs with the graphic interpretation structure grids from the geologist. We’ll talk with the reservoir engineers who are typically the ones who are trying to solve the problem of how to develop the section, ultimately responsible for the economic performance of a DSU. They’ll obviously have some ideas of how they want to complete these wells. They’ll also be the owners typically of the well level data such as production and well header.
“Petro.ai will pull all the client’s data together. This domain knowledge to sift through this data and a fair bit of work to get that data organized, cleaned up and processed.
“Then we’ll forecast the wells. That’s a reservoir engineering workflow that we do internally which we’ve automated. After that, we’ll characterize the stress profile. This is something that’s in the geosciences. It’s not really a geologist’s role. It’s more of a geophysicist or geomechanics expert. Oftentimes our clients will have access to this data but they aren’t actively using it. What they’re using is very qualitative.
“We’ve developed some workflows with Dr. Mark Zoback, Professor of Geoscience at Stanford University and Science Advisor for Petro.ai, for doing the stress profile development that has been not only tested in the lab, but also in the real-world work Petro.ai has done. We have validated the results in client after client. This is where Brendon spends a lot of his time and where Martin will be able to help as well.
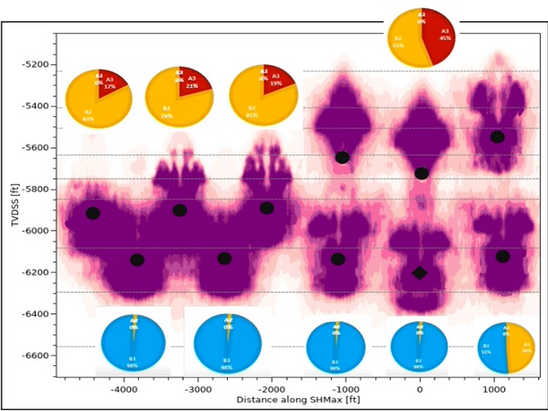
“From there, we’ll look at the Frac Fingerprint. We have a lot of models that we’ve already developed for basins. We can also tune that model with a variety of data types. That Frac Fingerprint responds to changes in stress. Frac Fingerprint really encapsulates geoscience characteristics with the stress profiles but also the different completion data types we’ll bring in to tune that or to calibrate the Frac Fingerprint.
“Then we have our feature pipeline. While we’ve automated the most repetitive parts of this workflow, it still requires domain knowledge to QC and interpret what’s happening. We’ll feed those features into the pipeline and generate the model with those features.
“Now we train the model. We look at feature correlations, building models, looking at cross validation accuracies. Our Petro.ai experts complement our customer asset teams by delivering leading-edge data science knowledge and best practices. Our team will be able to evaluate different models and understand good fits, bad fits, and what makes a model better or worse.
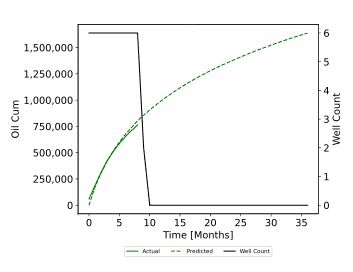
“We develop a series of models that we’ll work through and build. We’ll validate the performance with analog pads. The iterations on the model bring in economics and then ultimately make the prediction that the client is interested in seeing to help them make an informed decision. That could be a decision on how many wells to drill, where to put the wells, how to complete the wells, what order to complete the wells in, what could be the tradeoffs if they come back in six months or twelve months, and what the different parent child relationships will be at that time.