Q: What is a data driven methodology to predict the stress profile?
A: “Petro.ai wants to be as data driven as possible,” Kyle LaMotta, VP of Analytics explains in a recent interview, “Our core philosophy is to honor the data to reduce the variability and increase the efficiency of strategic decisions.”
“And we’re always updating our data driven methodologies with workflows that further automate significant parts of the process,” Dr. Brendon Hall, VP of Geoscience at Petro.ai adds. "That’s what’s happening right now at Petro.ai with the incorporation of Dr. Mark Zoback, Professor of Geoscience at Stanford University, Viscoplastic Stress Relaxation (VSR) Theory. This is hot off the presses and having a big impact on the report analysis that we provide to our clients.
“Basically, VSR states that in the clay rich ductile rocks, such as shales, the maximum and minimum horizontal stresses can get closer together over time creating a more uniform state. The effect being that the minimum horizontal stress will increase to meet the maximum horizontal stress.
“Let me give you some background for this understanding. Over the past several years Dr. Zoback has been working on the theoretical background for a data-driven approach to the stress profile calculation. Zoback’s new answer takes the subsurface data that companies collect which is predominately in the form of well logs and some advanced logging called sonic logs, where sonic logs measure the speed of sound in the earth that pressure waves travel. All these logs are used in combination with actual stress measurements of the minimum horizontal stress, from DFIT tests or ISIPs extracted from frac van files.
“Zoback uses those points to calculate a machine learning model which is a data driven approach that uses those well logs as well as those calibration points to predict a minimum horizontal stress using equations derived under the name of Viscoplastic Stress Relaxation (VSR) Theory.
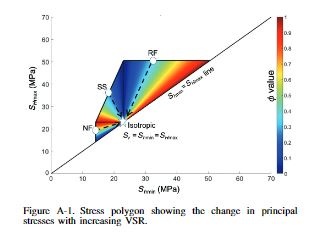
“Zoback has demonstrated this theory in the Midland basin using the HFTS1 data set.
Petro.ai has been applying this to some of our recent projects. With this concept, we built a highly accurate model for the Midland basin. This process has shown a high success rate for mapping these well logs to the stress measurements.
“The fundamental basis behind the VSR theory is that the rocks that we’re looking at in unconventionals like shale have a lot of clay in them. Shale also has a lot of organic material which is where the hydrocarbons come from. The clay and the organic material are different from quartz and calcium carbonate which are the fundamental constituents of classic rock or sandstone and carbonate rock. They are also very stiff components whereas the clay and organic pieces are not stiff.
“This means that the Young’s modulus can be lower. It used to be felt that the higher stress regions would just correspond to a higher Young’s modulus and rocks would be more brittle. On the other hand, over geologic time, rocks buried under the earth are always in a state of extreme stress. They’re being compressed by the weight of the rock above them. Tectonic forces are squeezing them together.
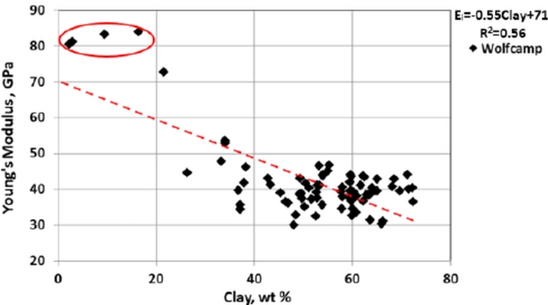
“So, the ductile rocks, the shales and rocks with high clay can relax over time. Stresses in those rocks can get closer together. Often, we talk about a maximum horizontal stress and a minimum horizontal stress.These, in these clay rich rocks, these ductile rocks, the maximum and minimum horizontal stresses can get closer together over time creating that more uniform state which is what the VSR Theory predicts. The effect that has on the minimum horizontal stress is that will increase to meet that maximum horizontal stress.
“When the minimum horizontal stress goes up that’s the control on the frac barrier. That’s why these ductile rocks could have a higher stress than can be predicted with other theories. It’s important to capture this effect and the VSR Theory is designed to do that.
“This new workflow is fully data driven in that it uses stress measurements, point measurements of stress in the earth as DFITs or ISIPs along with well logs to completely predict a stress profile. There’s a minimum of interpretation required if you have plenty of data to make that stress profile.”
“Exactly,” LaMotta finishes, “If we’re not relying on someone to interpret the results of some measurement then we can streamline the process. We calculate the stress profile using the VSR method and automatically run the drainage calculation from that instead of spending a week interpreting something and getting feedback. For us internally, this moves the Petro.ai reports quickly and efficiently forward making that an automated step which is highly accurate.”