Build: Building your own AI isn’t just about a data science team, it’s about recognizing that if you’re going to build an AI solution, you’re looking at many months, maybe even years of investment just to put together something that you’d call a prototype, never mind a functioning product. And that’s assuming you have a good team already in place and can communicate the information gaps you have.
Buy: Petro.ai minimizes the integration footprint with cloud deployment, a sophisticated data engine and more data types modeled than any other vendor in the AI space. With your data and proprietary information, the platform becomes your differentiator. Easy to plug in and ready to use.
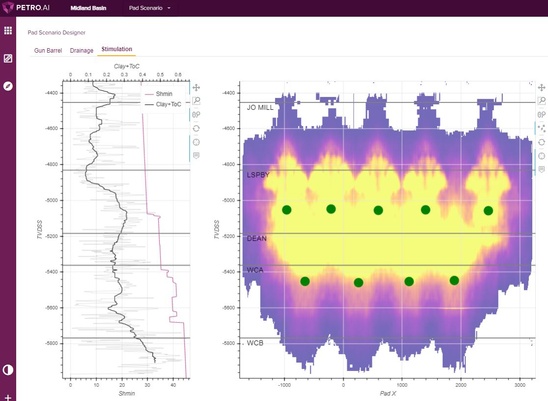
The days of in-house software development for the complex analysis needed by O&G companies are numbered. Even for super majors. AI, artificial intelligence, is the new differentiator. The buying rather than building of AI software will transform right out of the box, the functionality of your siloed users creating a broader multi-disciplined approach, support your resource factory focusing your skills on your core-competencies, and move your data science team to discussions of visualization analysis rather than software creation.
Buy versus Build
Dr. Derek Ruths, Chief Data Scientist of Petro.ai expands on the buy versus build, “One point that is important to raise, particularly from a CTO perspective, is there’s this perception of AI being a super heavy lift, very high risk, sort of dangerous. But that is only true for businesses that are trying to build their own AI solution. And to build in-house, they’re going to experience an enormous change management overhaul. You’re retooling your development team; you’re bringing in a data science team for the first time. You’re building new software.
“That’s very different from what Petro.ai is trying to do. Petro.ai is about bringing in a vetted solution that has AI in it.
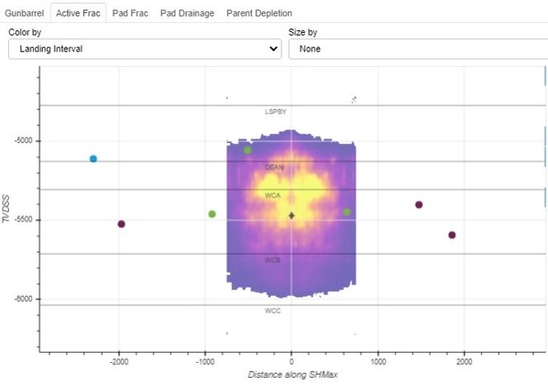
“I think the way we’re bundling AI in Petro.ai, is to give a company the power of AI without having to go through the AI journey. That’s the distinction between build versus buy. It is very complex to build AI. It’s a heavy investment and as a CTO you need to decide are you going to build an AI or are you going to have a vendor build an AI that you infuse with something? If there’s a software that comes off the shelf that works with your data, everybody is eventually going to do that because the advantages are so high.
“The final question becomes, can you really differentiate yourself with this AI once you buy it? Because that’s why you wanted to build it. From the Petro.ai perspective, we have easy to plug in places where if you’ve done some proprietary work, you can plug it into the AI in a very differentiated way. That would include the stratigraphic model, the stress model and the frac geometry model that can be customized.
“You start off with something that’s very easy to integrate into your environment. Then the more you integrate it, the more you make it proprietary to your needs and to your problem. Petro.ai becomes your differentiated platform.”
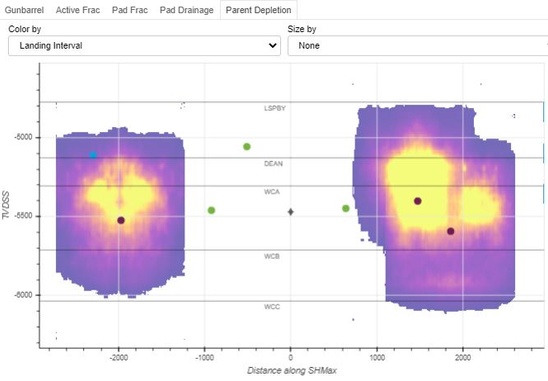
Multi-Disciplinary
“I’ve seen different siloed teams inside the same company, buy software for the same problem,” Dr. Troy Ruths, CEO of Petro.ai explains.“They’re trying to solve the same problem from different vantage points. Most interesting problems are multidisciplinary, especially in O&G. Well spacing, for instance, is a multidisciplinary problem. There’s a geo side to it, a reservoir side to it, there’s a completions side to it. So, what does it mean if you ask just the completions team to build an AI? You’re going to get a biased AI for completions.
“And from our perspective, the rock is the multidisciplinary challenge. It’s not just geo. We’re also trying to understand how the rock responds to stimulus. We’ve captured that with our apps and with the diverse set of data types that we pull in. We’re bringing in completions data, reservoir data, and geo data. That’s a very important part of the process and we have technical models that actually bridge across functional silos.
“How we break up Petro.ai is also multidisciplinary. We have Petro.ai the platform. And then we have models that live in Petro.ai that are the trained AI models. We have a composable environment with a platform, apps and models that allow us to grow with the value of the customer. We’ve helped make this a modular experience in rolling it out to your environment.”
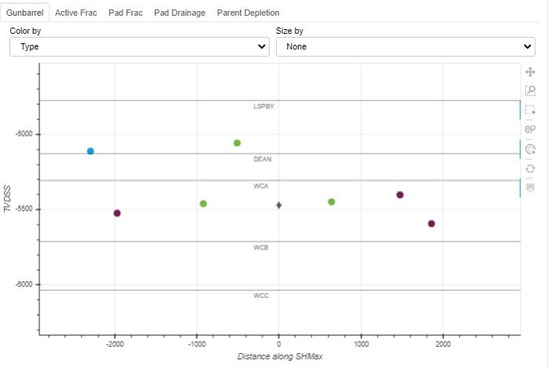
Resource Factory
Troy continues, “Our clients are not software companies. They are oil and gas companies discovering which rock to buy, exploring for reservoir, exploiting the reservoir. They are resource factories. The resource factory is still the key part of an O&G company. AI makes your resource factory more efficient. You’re using the latest and greatest tools in order to solve a business problem that you have. Petro.ai is a tool that your team can just pick up and use. You don’t have to cross that AI chasm.
“For operators, their differentiator is the rock that they have, the ability to technically model it and extract hydrocarbons in an economic way. They have zero infrastructure for developing a sophisticated AI. That’s not their core business. Some companies have analytics but zero AI. There is no operator that has applied AI as a differentiator.
“This was true back with seismic. We’re going to build seismic workstations and we’ll have a differentiator because we can look at seismic data. Now everyone has seismic workstations. We’re going to have a web mapping tool and now everyone has it. Don’t waste your time trying to be a software company. AI is a software. Your differentiator is how you manage that resource factory.”
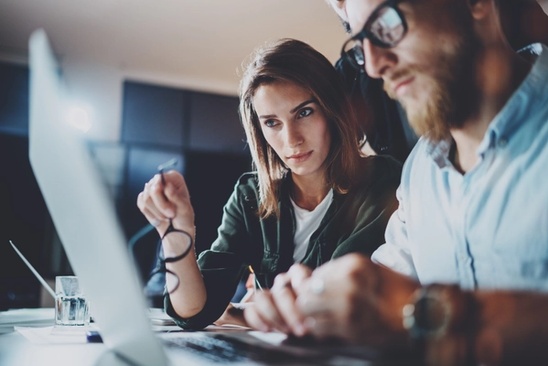
Data Science Teams
Yesterday’s Business Intelligence, BI, data science teams constructing analytics tools that are limited in scope and tethered to particular developers for long-past mission critical projects will be replaced by the use of revolutionizing Artificial Intelligence.
The Eckerson Group explains the transition from BI to AI tools for data scientists, “In the beginning, BI was mostly led by technical stakeholders who transformed data and presented insights in descriptive reports. But self-service BI requires that business users have basic knowledge about how to work with data and IT systems. Otherwise, they can easily come up with half-truths or become overwhelmed by possibilities. AI can help to overcome these issues by automatically supporting users, minimizing the degree of manual work, and introducing prescriptive features that not only predict what will happen but also recommend actions to take.”
For Troy this is an important distinction and use of talented data scientists.
“Your data science team should be helping with the resource factory. Data science teams should be getting the results of an AI like Petro.ai and applying that to a larger problem for their company, a higher value problem that’s unique to them.
“Have these trained engineers leverage the output of that AI as a tool in your data science stack. Have them ask, now that I can calculate drainage at scale very easily, what else could I solve? How could I apply the output at scale or in an automated way? Instead of saying, as companies large to small have done, how can we build a customized approach our own way? That’s a long road with no satisfactory end. We already have it. Buy it and apply it to optimizing your future.”