Optimization of a drilling spacing unit (DSU) with parent wells, or even the accurate forecast of infill wells, is hindered by the large degree of uncertainty around child well degradation.
Infill development wells have seen up to a 40% degradation from their parents in the Midland Basin.
Petro.ai demonstrates an AI workflow to predict infill well performance and determine optimal well placement that is applied to a five square mile development in the Midland Basin.
This workflow uses a flexible approach that accounts for various attributes influencing child well performance such as offset distance, cumulative production, or other features.
Engineers are able run design sensitivities on well count and placement to improve the economics of a development while reducing the uncertainty in their production forecasts.
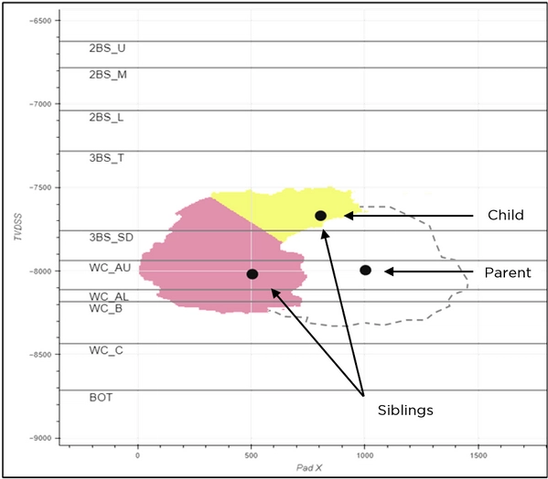
While the Frac Fingerprint methodolgy captures the primary drivers and first-order effects of parent/child interference, certain geographic regions do display a bias in over-predicting child wells due to higher-order effects beyond the scope of Frac Fingerprint assumptions (e.g. asymmetric lateral growth).
By training a meta-model to identify a Correction Factor (CF) and adding this feature to the model ensemble, prediction accuracies rose 30%.
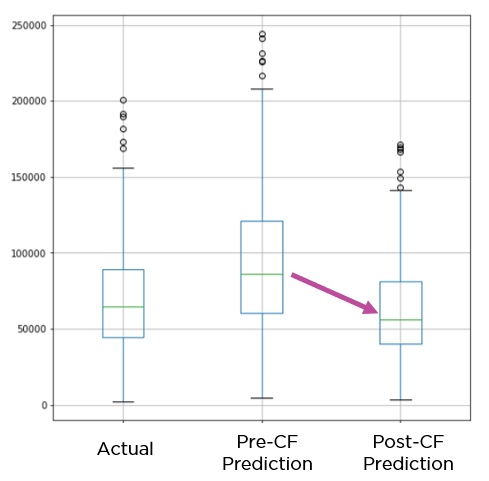
The final model, which included the derived CF, was then applied in a blind test to a recent 3-well pad brought on to production. The resulting blind predictions were within 15%, 2%, and 1% of actual production through 6 months of production.
We are looking forward to sharing the results in Denver on June 13. Our other publications and presentations can be found here.